1. Focus on Critical Machinery and Equipment: It is essential to start the PdM program by identifying and prioritising assets that have incurred the most substantial losses. This ensures that maintenance efforts are focused on critical areas first.
2. Gradual Implementation: Start with pilot projects on critical equipment and gradually extend the program, learning and adapting as the process evolves. This allows for a more manageable analysis of the predictive maintenance model and its impact before scaling up.
4. Regular Workforce Training: Engage and train your workforce to adapt to new predictive maintenance technologies, ensuring they are capable of utilising these systems effectively.
5. Tailored Predictive Models: Customise predictive maintenance models to suit the specific equipment and environmental conditions of the mining operations.
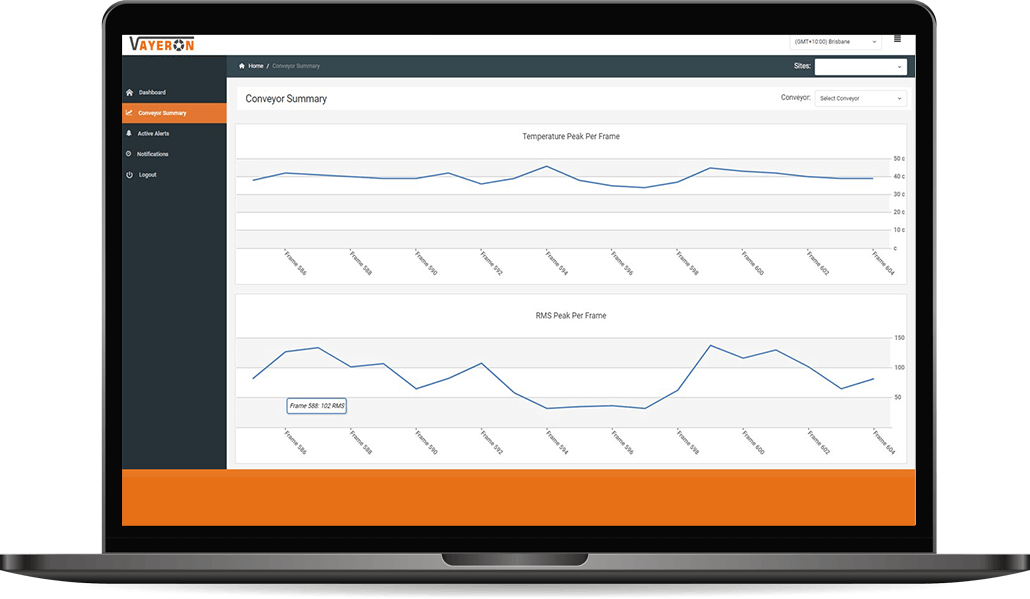
7. Continuous Review and Improvement: Regularly review and update PdM strategies based on new data, insights, and technological advancements.
8. Collaboration with Tech Partners: If in-house expertise is lacking, consider collaborating with outside vendors, experts or consultants who specialise in predictive maintenance. They can offer valuable guidance throughout the implementation process and provide ongoing support.
9. Change Management: A systematic approach to managing the organisational change associated with the implementation of new technologies is vital for the successful adoption of predictive maintenance strategies.
Applications of Predictive Maintenance Practices
In the mining industry, best practices and strategies in predictive maintenance play a crucial role in maintaining the reliability of heavy machinery, such as crushers, conveyor belts, and excavators. By preventing unexpected breakdowns, they help minimise costly downtime and maintain production targets.
Examples from the Mining Industry
- BHP: BHP has been utilising the best practices in predictive maintenance to monitor the health of their large mining equipment, significantly reducing downtime and maintenance costs. By leveraging data analytics, BHP has been able to predict equipment failures, schedule maintenance more effectively, and improve overall operational efficiency.
- Rio Tinto: Rio Tinto’s deployment of PdM technologies has enabled the company to optimise its maintenance schedules, leading to increased equipment uptime and reduced operational costs. Their advanced data analytics capabilities have played a pivotal role in predicting and preventing equipment failures.
Downtime for Rio Tinto’s enormous haul trucks, which haul iron ore around the clock, translates directly to lost revenue. A single day out of service costs a hefty $2 million, and that price tag jumps even higher for tow-away situations. To combat this expensive issue, Rio Tinto embraced the power of the Internet of Things (IoT) for predictive maintenance. The company outfitted its fleet of 900 dump trucks with a whopping 92 sensors each. These sensors constantly monitor engine, drivetrain, and wheel performance, generating a daily data stream of a staggering 4.9 terabytes. Using advanced computer algorithms, including deep learning, this data is analysed in near real-time. The system can, for example, pinpoint a 60% chance of a part failing within three weeks. With this foresight, Rio Tinto can proactively order parts and schedule maintenance, drastically reducing unplanned downtime and saving significant costs.
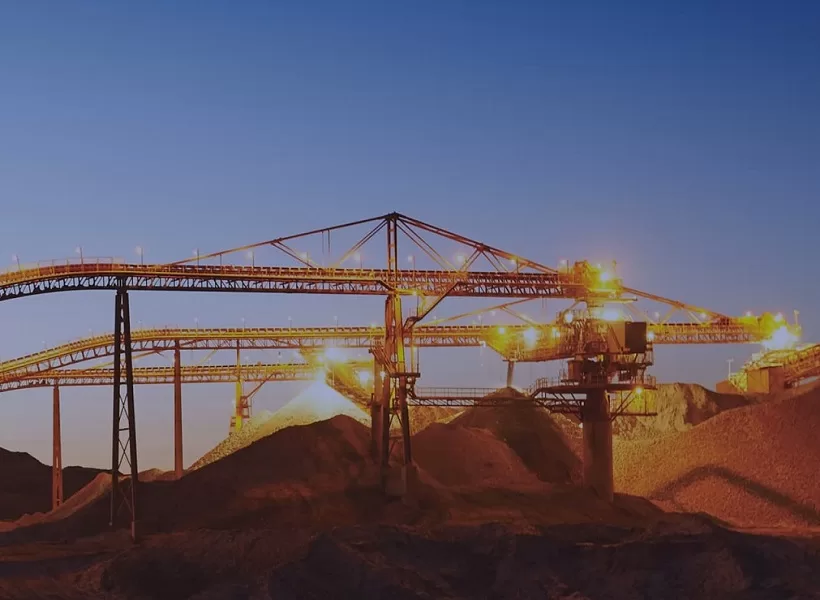
- Newcrest Mining: Newcrest’s implementation of predictive maintenance technologies, particularly in their conveyor belt systems, has resulted in a noticeable decrease in unscheduled maintenance and equipment breakdowns, enhancing productivity and safety in their operations.
- Newmont: Newmont has embraced PdM for their mining vehicles and machinery, utilising a combination of IoT and AI to monitor equipment health. This proactive approach to maintenance has resulted in improved equipment availability and reduced maintenance expenses.